For all arguments, data sources matter, and some broad assumptions influence how Audiences and Speakers consider sources. University sources of information are generally among the most respected of all sources. Many consider governmental agencies and scientific groups as authoritative sources. Industry, trade associations, non-governmental organizations (NGOs) and watchdog groups can also provide accurate and robust data, but they might have different agendas than impartially reporting data. Anecdotal data from a friend or family member can be emotionally compelling and true for that case, but may not provide a representative example suitable for a reasoned argument.
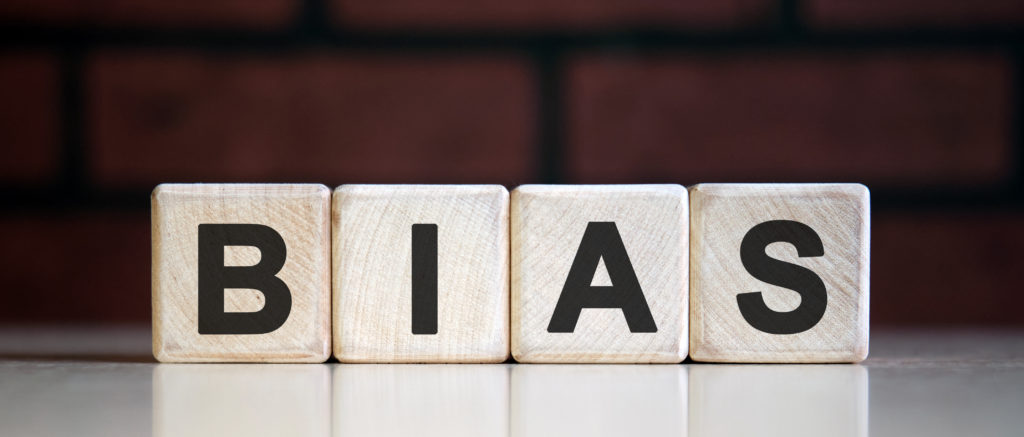
Evaluating the stakeholders behind any data source is crucial to identifying the quality of the source and any bias that might be explicitly or implicitly retained within. Every data set and argument has a bias, because no individual or group can be unbiased. Further, while all groups have inherent biases, some promote a specific agenda, which can affect the data they release.
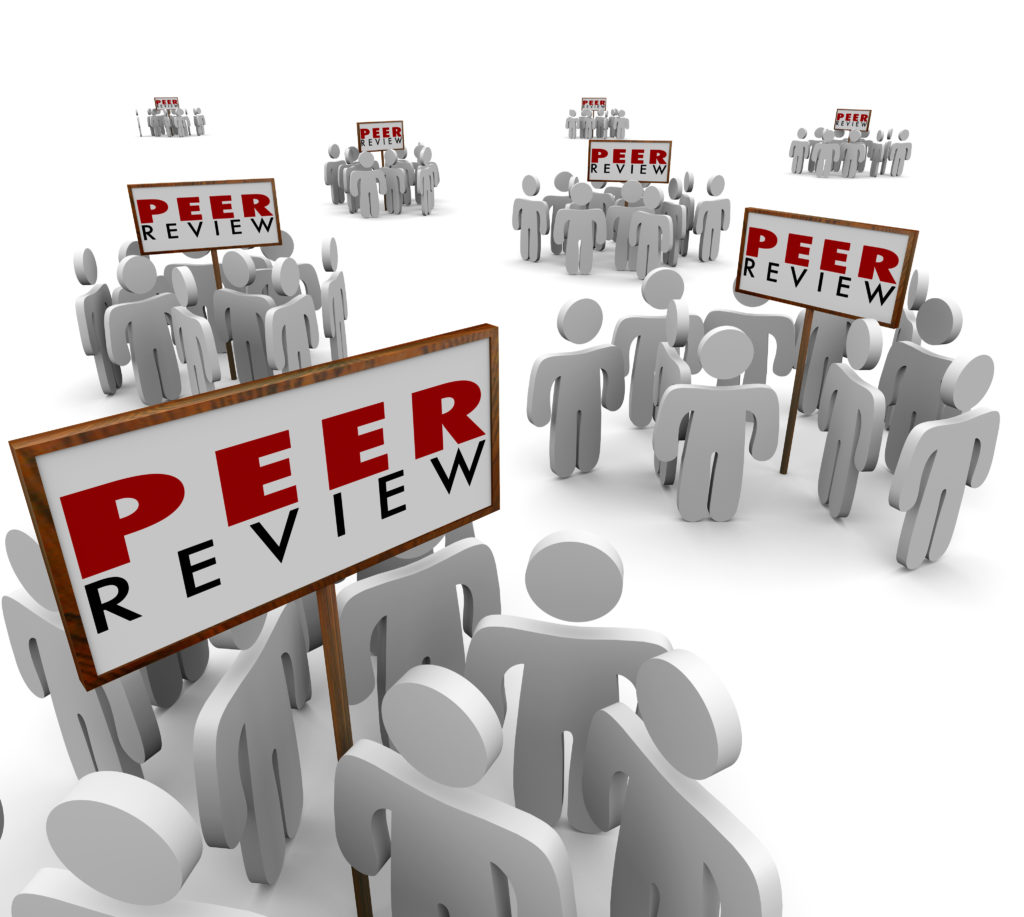
In addition, it is worth examining whether the data were subjected to peer review or some other verification process. Some well-respected and widely cited energy data sets are included in the Annual Energy Outlook or Monthly Energy Review from the U.S. Energy Information Administration (EIA) or the World Energy Outlook from the International Energy Agency (IEA).
Sources do not always agree due to differences in data collection, assumptions and analysis, thus Speakers crafting data-informed arguments take on the challenge of evaluating different sources and using them together. They also have the unique challenge of judging whether or not the biases or agendas of a particular dataset strengthen or detract from a particular argument.
If a research paper about induced seismicity used only one data source derived from an oil and gas company, readers would likely suspect a bias in favor of continued operations. Similarly, if the same paper only used one data source from a highly vocal “anti-fracking” NGO, readers would suspect that the data was biased in favor of banning hydraulic fracturing outright. A paper that takes data from multiple sources with different biases and synthesizes the data to support a hypothesis is most likely to be perceived as impartial and accurate by all who read it.
Images: “Business graph” by Who is Danny via Shutterstock; “Bias” by Kavaleuskaya Aksana via Shutterstock; “Peer Review” by iQoncept via Shutterstock